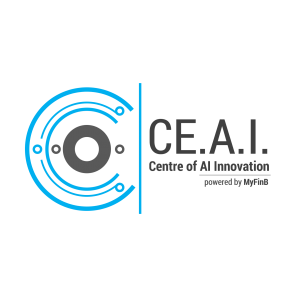
"Can artificial intelligence be deployed to slow down global warming, or is AI one of the greatest climate sinners ever? That is the interesting debate that finds (not surprisingly) representatives from the AI industry and academia on opposite sides of the issue."
Can artificial intelligence be deployed to slow down global warming, or is AI one of the greatest climate sinners ever? That is the interesting debate that finds (not surprisingly) representatives from the AI industry and academia on opposite sides of the issue.
While PwC and Microsoft published a report concluding that using AI could reduce world-wide greenhouse gas emissions by 4 percent in 2030, researchers from the University of Amherst Massachusetts have calculated that training a single AI model can emit more than 626,000 pounds of carbon dioxide equivalent—nearly five times the lifetime emissions of the average American car. Who is right?
The big players have clearly understood that the public sensibility towards climate change offers a wonderful marketing opportunity. IBM has launched its Green Horizons project to analyze environmental data and predict pollution. Google has announced having cut by 15 percent the amount of energy used in its data centres thanks to machine learning. Forecasting energy consumption, analyzing satellite data to monitor forest conditions in real time are additional examples. The periodical announcement of new applications of AI that might help fight global warming are meant to put green cloths on an industry that, in fact, consumes devastating amounts of energy. This diverts our attention from the question that really matters:
How to reduce the carbon footprint of AI?
I already emphasized it in my piece “Brute force is not the answer“: the race for better performing supercomputers is not going in the direction of a sustainable AI. Supercomputers are demanding beasts that not only need a lot of space (the equivalent of two tennis courts for IBM’s Summit), but also require millions of gallons of water to cool down and consume as much power as 7,000 households.
Which alternatives do we have?
Alternative 1: Quantum Computing
Quantum computing is the ability to transform the classical memory state of a computer (distinct series of 0 and 1) into a superposition state that enables an unprecedented level of parallel calculations. In theory, a quantum computer is exactly what is needed to accelerate AI tasks. That is the reason why all major companies and academic groups work hard on testing, refining and reproducing quantum computing models. The expectations are very high: quantum computers should be able to process within seconds what a standard computer would take several years to analyze. But, despite the huge efforts put into this research field and the decades of experimentation, no-one has ever seen a quantum computer capable of performing a real-world task. And most experts agree that the time horizon to implement quantum AI applications is… very long.
Alternative 2: Accelerating Hardware
Your mobile phone is full of them, your computer is full of them, your game console is full of them: Graphic Processing Units (GPUs) are ubiquitous when it comes to processing large blocks of data like images or videos in parallel. But there is much more going on than GPUs. In answer to the demand for high speed processing hardware, a plethora of new AI-optimized chipset architectures have been introduced in the past years, such as neural network processing units (NNPUs), field programmable gate arrays (FPGAs), or application-specific integrated circuits (ASICs). Besides the core AI chipsets manufacturers Nvidia, Xilinx and Intel, major tech companies like Microsoft, Google, Amazon, Apple and Tesla are also working on their own AI processors. And more and more start-ups are trying to seize their share of the booming AI-chips market. There is so much offer meanwhile for AI-accelerator chips that vendors of AI computing platforms combine different chips and that it is difficult to find the best architecture for one’s project.
Alternative 3: Reverse-Engineering the Brain
While our own intelligence needs a mere 20 watt to reason, analyze, deduct and predict, an artificial intelligence system like IBM Watson needs 1,000 time more power to perform complex calculations. A logical step is to try to replicate the wiring of the brain to reduce the computing power needed by AI processes. From a hardware perspective, this has been attempted with the development of “neuromorphic chips“. But, if researchers have found how to replicate the structure of the brain in a processor, they are still at odds about how to emulate how it works.
This means that a new processing model is needed, a piece of software that learns and understands information like the brain does. A system that does not need to be fed with thousands of examples to learn a new concept, or a completely new setup when the parameters evolve. This quest towards machine intelligence is one of the most demanding scientific challenges of all time, to quote Numenta, a Silicon Valley-based company which has dedicated its efforts to solving this problem. Numenta’s framework replicates the way our brains process information. Applied to Natural Language Understanding, this new form of machine intelligence enables high performing business applications that need little training and computing power, even when implemented with general-purpose processors. Combined with accelerating hardware, the gains in terms of speed and savings in terms of energy consumption will be tremendous.
There is no doubt that, in times where a sixteen-year-old green activist from Sweden makes the headlines and masses of young people block the streets every Friday, the AI industry cannot afford being accused of massively contributing to global warming. Polishing its image with anecdotal stories of hypothetical green applications will not be enough. What the AI sector needs is a paradigm shift, away from the current CPU race towards a responsible, sustainable and economical processing framework. First initiatives have started. They are by far not enough.